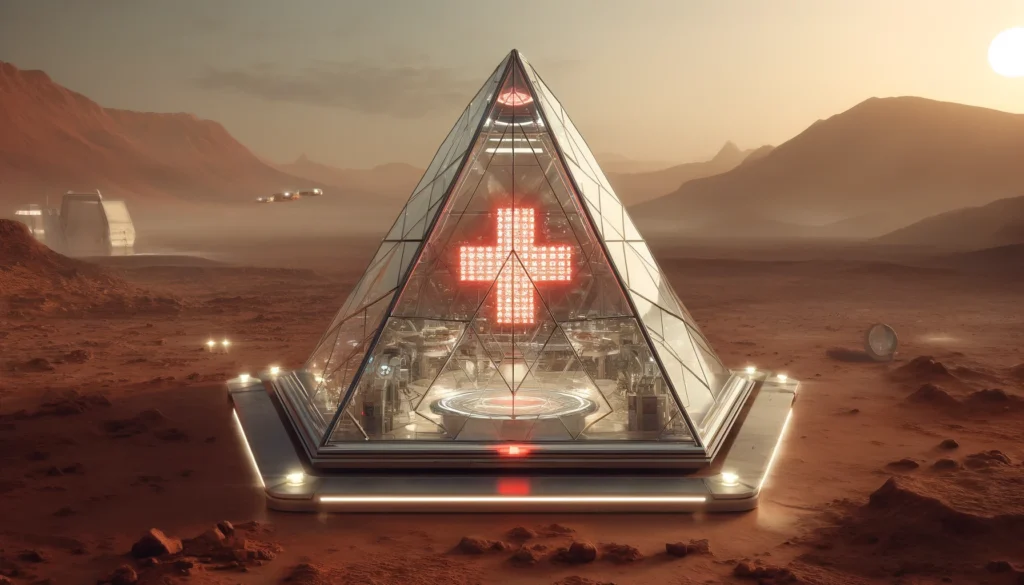
Models like BioBERT, ClinicalBERT, and PubMedGPT are making waves. They’re fine-tuned on medical texts, making them sharp tools for healthcare tasks.
- BioBERT: This model, trained on biomedical literature, shines in tasks like named entity recognition (NER) and relation extraction [1].
- ClinicalBERT: Tailored for clinical notes from EHRs, ClinicalBERT excels in predicting patient outcomes and spotting medical conditions [2].
- PubMedGPT: Based on GPT-3 and trained on PubMed, it generates clear, relevant medical text for documents and research summaries [3].
Next, we have multimodal models. These beauties integrate text with images and structured data, boosting diagnostic accuracy and personalized treatment plans.
- Med-T5: This model combines text with radiology images, offering accurate diagnoses and recommendations [4].
Making these models understandable is key. Researchers are on it.
- Attention Mechanisms: These highlight relevant parts of the text, helping clinicians grasp the model’s predictions [5].
- Post-hoc Explanation Tools: Tools like LIME and SHAP explain individual predictions, giving insights into the model’s decisions [6].
Benchmarks
Datasets
Medical LLMs are put to the test on benchmark datasets:
- MIMIC-III: Used for tasks like predicting patient outcomes and recognizing clinical entities [7].
- BioASQ: Assesses models’ ability to retrieve and summarize biomedical information [8].
- MedQA: Evaluates how well models answer complex medical questions [9].
Key metrics include accuracy, F1 score, ROUGE score, and BLEU score.
Results
Recent research shows impressive results:
- BioBERT: Achieved an F1 score of 84.7 on the NER task in the BioCreative V CDR dataset [10].
- ClinicalBERT: Improved patient readmission rate predictions by 10% [11].
- PubMedGPT: Generated summaries with a ROUGE-1 score of 45.6 on PubMed abstracts [12].
Applications in Healthcare
Clinical Decision Support
Medical LLMs are game-changers in clinical decision-making:
- Diagnosis Assistance: Integrated into EHR systems, they flag potential diagnoses from clinical notes [13].
- Treatment Recommendations: Analyze patient history and symptoms to suggest personalized treatments, reducing risks and improving outcomes [14].
Medical Research
LLMs speed up medical research by processing massive amounts of literature:
- Literature Review Automation: Tools like PubMedGPT scan and summarize research articles, helping researchers stay updated [15].
- Hypothesis Generation: These models analyze data to suggest new research areas [16].
Patient Interaction
LLMs enhance patient interaction through chatbots and virtual assistants:
- Symptom Checkers: AI-powered checkers evaluate symptoms and suggest possible conditions and actions [17].
- Virtual Health Assistants: Offer 24/7 support, answering questions, scheduling appointments, and managing medication reminders [18].
Challenges
- Protecting patient data is crucial. Researchers are exploring ways to anonymize data and strengthen security.
- Addressing biases in training data to ensure fair healthcare delivery is vital [19].
- Finally, continuous learning mechanisms are being developed to keep LLMs updated with the latest medical knowledge and guidelines [20].
References
- BioBERT: A Pre-trained Biomedical Language Representation Model for Biomedical Text Mining
- ClinicalBERT: Modeling Clinical Notes and Predicting Hospital Readmission
- PubMedGPT: A Generative Pre-trained Transformer for Biomedical Text
- Med-T5: A Transformer-Based Model for Multimodal Medical Data
- Explaining Neural Networks in Clinical NLP
- Post-hoc Interpretability for Neural NLP: A Survey
- MIMIC-III: A Free, Open Critical Care Database
- BioASQ: A Challenge on Large-Scale Biomedical Semantic Indexing and Question Answering
- MedQA: A Benchmark for Medical Question Answering
- Evaluating BioBERT on Biomedical Named Entity Recognition Tasks
- ClinicalBERT: Predicting Clinical Outcomes with Pre-trained Language Models
- PubMedGPT: Benchmarking Biomedical Text Summarization
- Clinical Decision Support with ClinicalBERT
- AI for Personalized Treatment Recommendations in Healthcare
- Automating Literature Reviews with PubMedGPT
- Hypothesis Generation in Medical Research Using LLMs
- AI-Powered Symptom Checkers: Transforming Patient Interaction
- Virtual Health Assistants: Enhancing Patient Support
- Addressing Bias in Medical AI
- Continuous Learning for Medical LLMs